Breadcrumb
Computer aided diagnosis system for classification of microcalcifications in digital mammograms
Breast cancer is the main cause of death for women between the ages of 35 to 55. Mammogram breast X-ray is considered the most reliable method in early detection of breast cancer. Microcalcifications are among the earliest signs of a breast carcinoma. Actually, as radiologists point out, microcalcifications can be the only mammographic sign of non-palpable breast disease which are often overseen in the mammogram. In this paper a method is proposed to develop a Computer-Aided Diagnostic system for classification of microcalcifications in digital mammograms, it splits into three-step process
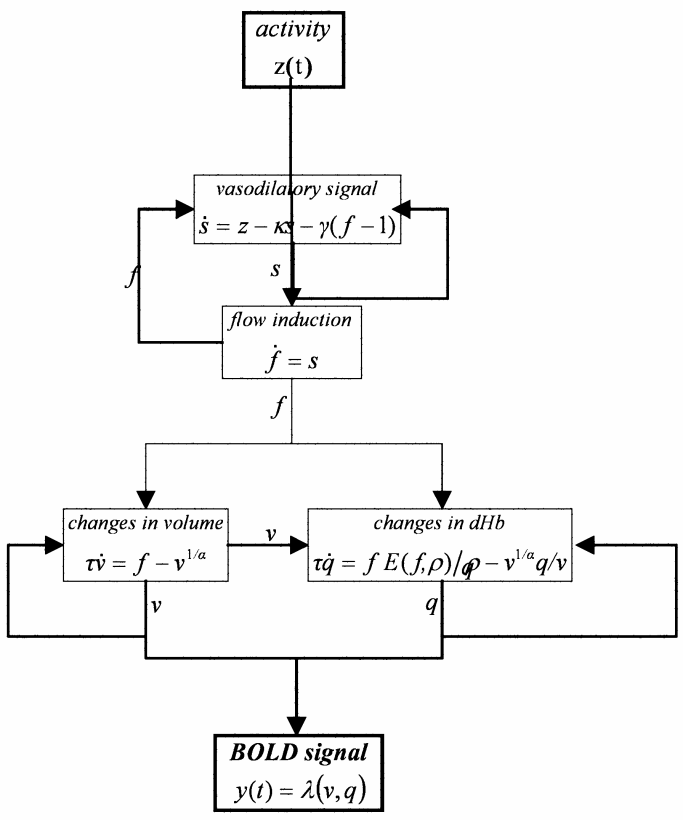
Modeling the interaction of brain regions based on functional magnetic resonance imaging time series
We propose a model that describes the interaction of several Brain Regions based on Functional Magnetic Resonance Imaging (FMRI) time series to make inferences about functional integration and segregation within the human brain. The method is demonstrated using dynamic causal modeling (OeM) using real data to show how such models are able to characterize interregional dependence. We extend estimating and reviewing designed model to characterize the interactions between regions. A further benefit is to estimate the effective connectivity between these regions. All designs, estimates, reviews
Different regions identification in composite strain-encoded (C-SENC) images using machine learning techniques
Different heart tissue identification is important for therapeutic decision-making in patients with myocardial infarction (MI), this provides physicians with a better clinical decision-making tool. Composite Strain Encoding (C-SENC) is an MRI acquisition technique that is used to acquire cardiac tissue viability and contractility images. It combines the use of blackblood delayed-enhancement (DE) imaging to identify the infracted (dead) tissue inside the heart muscle and the ability to image myocardial deformation from the strain-encoding (SENC) imaging technique. In this work, various machine
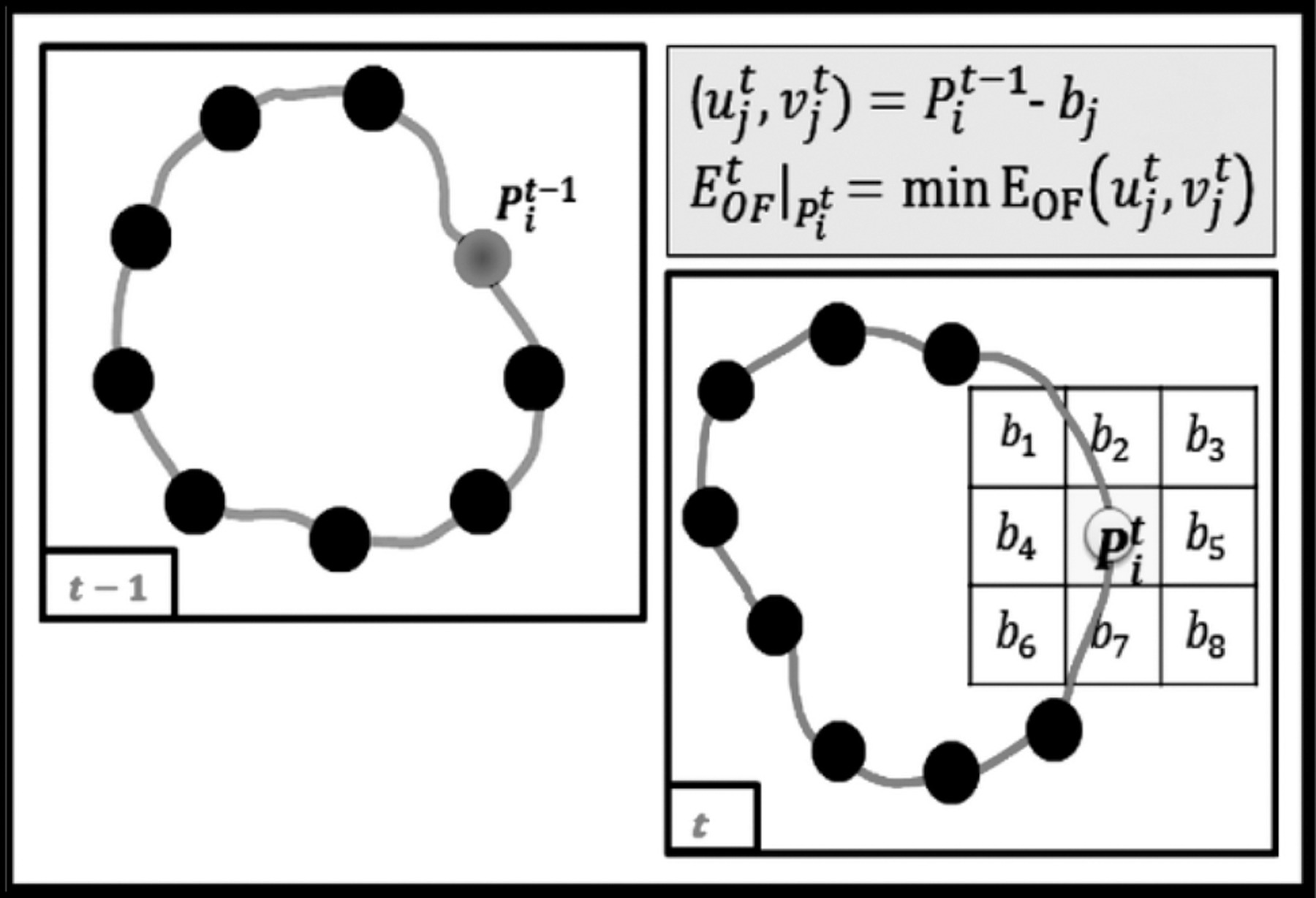
Greedy framework for optical flow tracking of myocardium contours
Optical flow (OF) tracking of the myocardium contours has a potential in segmenting the myocardium in time sequences of cardiac medical images. Nevertheless, to estimate the displacement field of the contour points, a number of assumptions are required to solve an under-determined set of optical flow equations. In this work, a new framework is proposed to solve the OF tracking problem using greedy optimisation algorithm. The new framework allows different types of constraints such as motion invariance, shape and topology to be applied in a unified way. The developed methods are applied to a
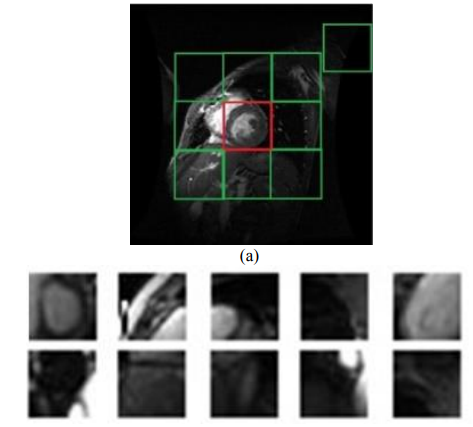
Automatic localization of the left ventricle in cardiac MRI images using deep learning
Automatic localization of the left ventricle (LV) in cardiac MRI images is an essential step for automatic segmentation, functional analysis, and content based retrieval of cardiac images. In this paper, we introduce a new approach based on deep Convolutional Neural Network (CNN) to localize the LV in cardiac MRI in short axis views. A six-layer CNN with different kernel sizes was employed for feature extraction, followed by Softmax fully connected layer for classification. The pyramids of scales analysis was introduced in order to take account of the different sizes of the heart. A publically
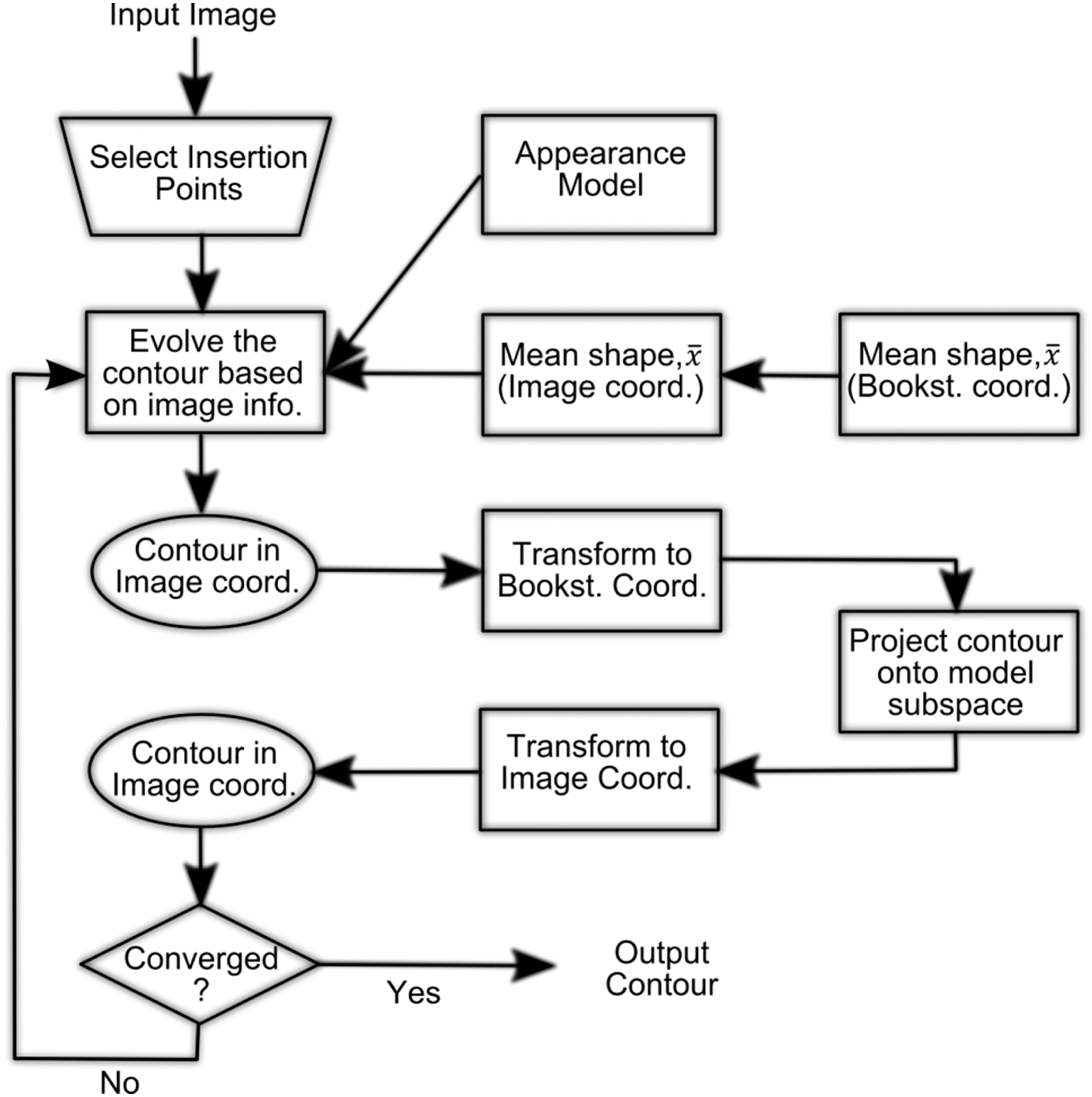
Segmentation of the right ventricle in MRI images using a dual active shape model
Active shape models (ASM) showed to have potential for segmenting the right ventricle (RV) in cardiac magnetic resonance images (MRIs). Nevertheless, the large variability and complexity of the RV shape do not allow for concisely capturing all possible shape variations among patients and anatomical cross-sections. Noticeably, the latter increases the number of iterations required to converge to a proper solution and reduces the segmentation accuracy. In this study, the authors propose a new ASM framework that can model the RV shape in short-axis cardiac MRI images. In this framework, the RV
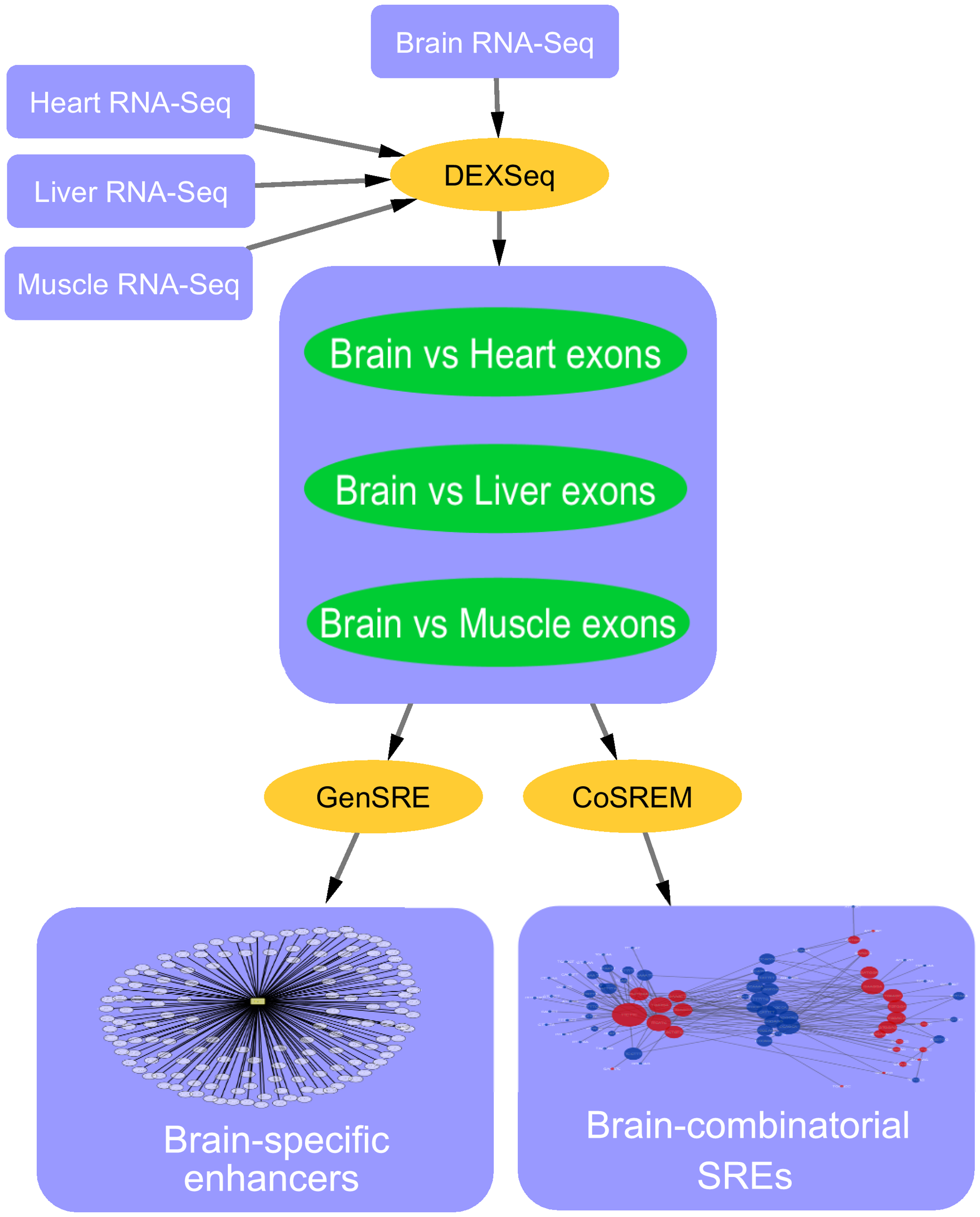
Computational identification of tissue-specific splicing regulatory elements in human genes from RNA-Seq Data
Alternative splicing is a vital process for regulating gene expression and promoting proteomic diversity. It plays a key role in tissue-specific expressed genes. This specificity is mainly regulated by splicing factors that bind to specific sequences called splicing regulatory elements (SREs). Here, we report a genome-wide analysis to study alternative splicing on multiple tissues, including brain, heart, liver, and muscle. We propose a pipeline to identify differential exons across tissues and hence tissue-specific SREs. In our pipeline, we utilize the DEXSeq package along with our previously
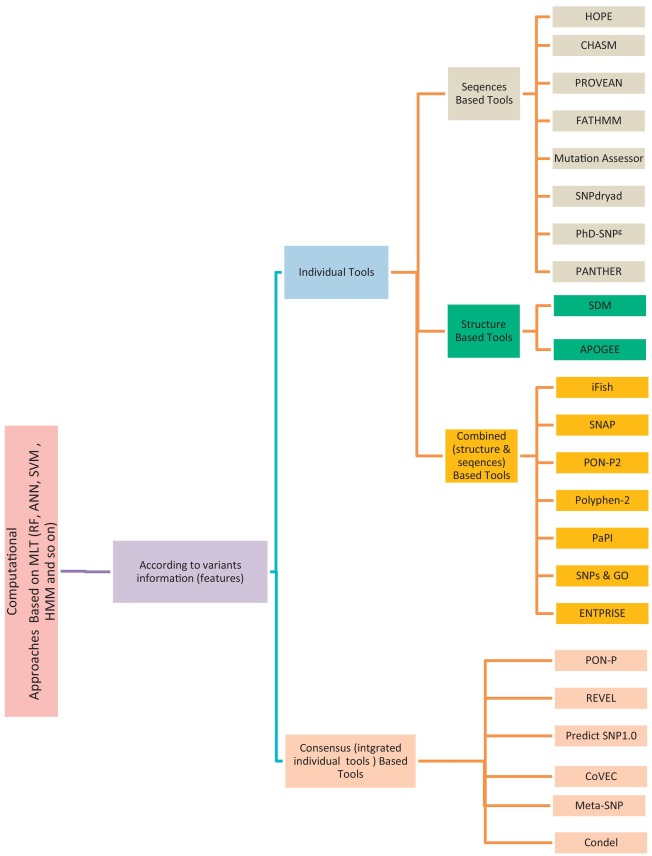
A review study: Computational techniques for expecting the impact of non-synonymous single nucleotide variants in human diseases
Non-Synonymous Single-Nucleotide Variants (nsSNVs) and mutations can create a diversity effect on proteins as changing genotype and phenotype, which interrupts its stability. The alterations in the protein stability may cause diseases like cancer. Discovering of nsSNVs and mutations can be a useful tool for diagnosing the disease at a beginning stage. Many studies introduced the various predicting singular and consensus tools that based on different Machine Learning Techniques (MLTs) using diverse datasets. Therefore, we introduce the current comprehensive review of the most popular and recent
Towards scalable and cost-aware bioinformatics workflow execution in the cloud - Recent advances to the tavaxy workflow system
Cloud-based scientific workflow systems can play an important role in the development of cost effective bioinformatics analysis applications. So far, most efforts for supporting cloud computing in such workflow systems have focused on simply porting them to the cloud environment. The next due steps are to optimize these systems to exploit the advantages of the cloud computing model, basically in terms of managing resource elasticity and the associated business model. In this paper, we introduce new advancements in designing scalable and cost-effective workflows in the cloud using the Tavaxy
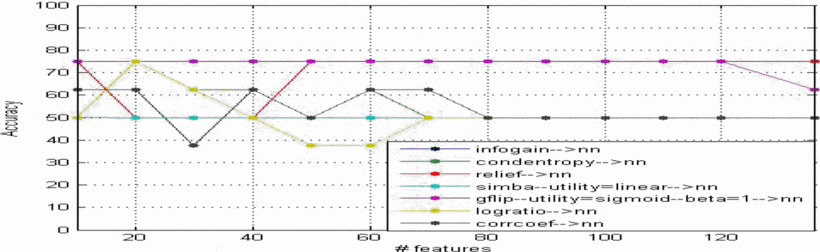
Feature selection in computer aided diagnostic system for microcalcification detection in digital mammograms
In this paper an approach is proposed to develop a computer-aided diagnosis (CAD) system that can be very helpful for radiologist in diagnosing microcalcifications' patterns in digitized mammograms earlier and faster than typical screening programs and showed the efficiency of feature selection on the CAD system. The proposed method has been implemented in four stages: (a) the region of interest (ROI) selection of 32x32 pixels size which identifies clusters of microcalcifications, (b) the feature extraction stage is based on the wavelet decomposition of locally processed image (region of
Pagination
- Previous page ‹‹
- Page 5
- Next page ››