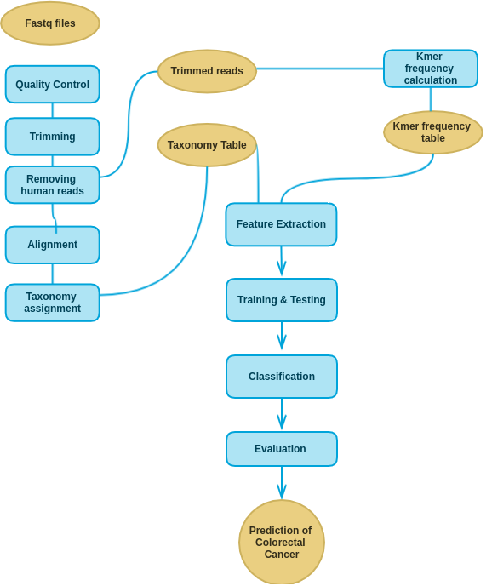
A Hybrid Machine Learning Approach for the Phenotypic Classification of Metagenomic Colon Cancer Reads Based on Kmer Frequency and Biomarker Profiling
Human Microbiome plays a critical role in health and the environment. Colorectal cancer (CRC) is the most common cause of death in many countries, and hence early diagnosis of CRC may help in increasing the survival rate. Tracking changes in the microbiome structure of human gut opens new gates towards the detection and prediction of the risk of CRC. Recently, machine learning became a powerful technique in many bioinformatics fields, one of which is metagenomics. Metagenomics is defined as the study of a collection of microbial genomes isolated directly and sequenced from its natural habitats. Applications of machine learning in metagenomics are numerous, among them are phenotype classification, taxonomic assignment, and sequence annotation. Phenotype classification is assigning a phenotypic class to each sample such as diseased or healthy, according to the available metadata. Phenotype classification in metagenomics is usually done on organism taxonomic units (OTU) tables extracted as a core step in the metagenomic analysis. On the other hand, Natural Language Processing (NLP) methods such as kmer frequency, can provide features for the machine learning model. In this study, we combined a biomarker profiling and a kmer frequency table approaches to classify colorectal cancer from metagenomic data. © 2018 IEEE.