Image
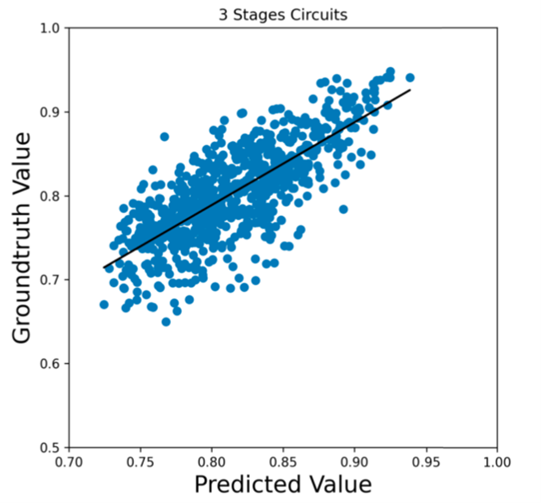
Practical Quantum Neural Network Computation on Noisy, Intermediate Scale-Quantum Computers
Practical Quantum Neural Network Computation on Noisy, Intermediate Scale-Quantum Computers
PI of the project: Prof. Ahmed Hazem El-Mahdy
Funded by:
- STDF
- E-JUST
- Waseda
Highlights:
Fidelity Estimation:
- Estimating the Fidelity is a very complex task, requiring an exponential number of experiments
- We showed that we can learn the fidelity using CNN with polynomial complexity
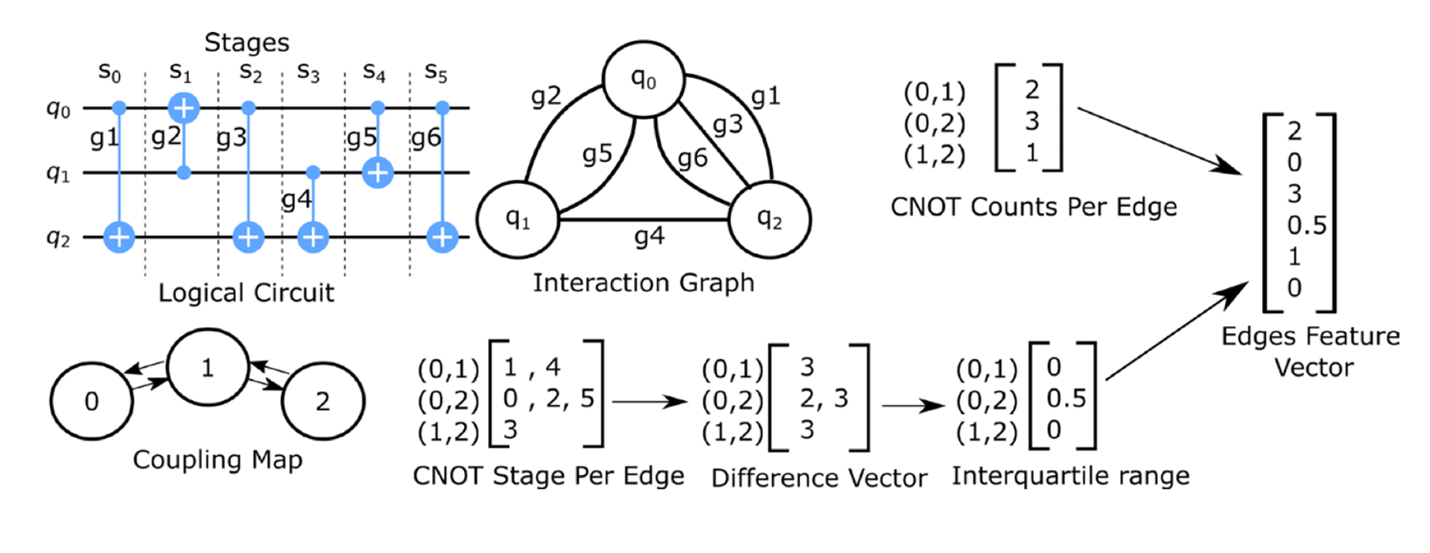
Improving Fidelity:
- The way quantum circuits are mapped into physical qubits significantly affects their fidelity
- We used reinforcement learning to reach a ‘good’ map
- We are comparable by the state-of-the-art but our approach is much faster:
- More than 10 times reduction on the feature-length size!
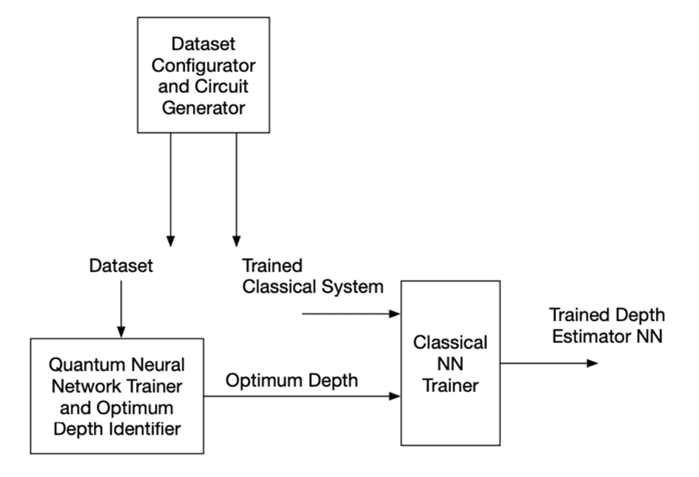
Circuit Parameterisation for Quantum Machine Learning:
- QNN dramatically reduces the number of parameters compared to classical
- An important issue is the number of parameters, need to avoid:
- Overparameterisation
- Underparameterisation
- We estimate the number of layers in the QNN given a standard NN
- We achieve 86.7% accuracy